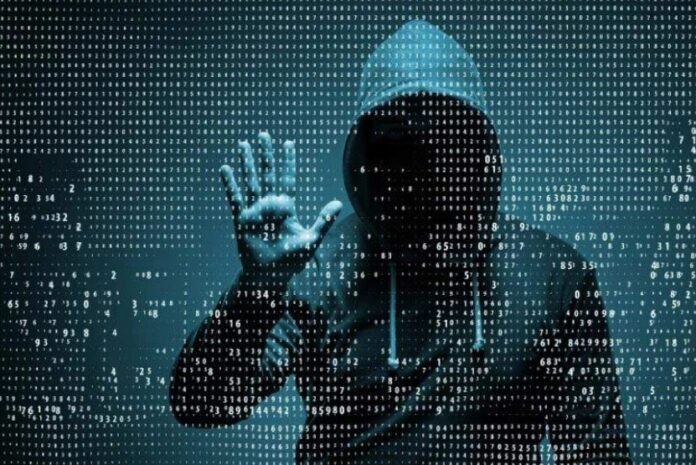
Artificial Intelligence (AI) algorithms play a vital role in enhancing their capabilities in contemporary technology, including security systems and devices. An algorithm is a set of instructions guiding a computer or system to perform a specific task. Security technologies leverage AI algorithms to carry out a range of functions. These include pattern and signal identification, such as recognizing acoustic signals produced by gunshots. AI can also detect anomalies in behavioral patterns through surveillance systems, differentiate between individuals and animals using computer vision, or identify and flag images or materials, like contraband or substances, in X-ray scanners.
The integration of AI in security technologies brings about significant benefits in terms of operational security. It enhances the probability and speed of detection, reduces operators’ workload and fatigue, and aids in directing the attention of security personnel to critical areas. Furthermore, at a management level, AI can lead to cost reduction, optimize resource allocation, support decision-making processes, and even present early intervention opportunities to mitigate insider threats.
It’s important to note that many security technologies utilize AI at a basic level to accomplish specific tasks. While some technologies may appear to possess a higher level of intelligence due to their ability to perform multiple tasks simultaneously, the level of intelligence is not solely determined by the number of tasks performed. Rather, intelligence levels are more closely associated with the complexity and integration of decision-making processes within a system or device.
In conclusion, the use of AI in security technologies brings numerous advantages. It enhances operational security, streamlines processes, and offers valuable support to security professionals and management. By leveraging AI, security systems and devices become more efficient, proactive, and effective in safeguarding assets and mitigating risks.
Current Use of Artificial Intelligence in Security Technologies
Observation, detection, controlling, and response technologies are integral components of security systems. Each technology category utilizes a combination of Symbolic and Statistical AI Paradigms, with specific elements aligned to different paradigms based on their functionality. The advancement of AI in security technologies presents opportunities for enhanced threat detection, efficient response mechanisms, and improved asset protection.
Observation Technologies, as defined by ASIS International, play a crucial role in detecting and characterizing potential threats, aiding in response formulation, and assisting in post-event investigations. Network surveillance systems are a prominent example of observation technologies and are categorized under Symbolic and Statistical AI Paradigms. These systems encompass various components, such as video analytics and management systems, which align with Symbolic and Statistical Paradigms. Drones and robotic sensors also fall under Symbolic AI, with analytics and management systems spanning Symbolic and Statistical Paradigms. It’s worth noting that, currently, there are no Observation technologies within the Sub-symbolic AI Paradigm.
Detection Technologies are integral to Intrusion Detection Systems (IDS) and Physical Protection Systems (PPS). These technologies are utilized to identify unauthorized actions and communicate alarms to control centers for assessment. Detection technologies encompass a range of sensors, alarm devices, lighting systems, x-ray technology, and more. Examples of detection technologies within the Symbolic and Statistical AI problem domains include intrusion detection systems, biometric systems, SONAR and RADAR sensors, network surveillance video analytics, acoustic detection systems, drone sensors, x-ray sensors, trace detection systems, and lighting systems. Each technology aligns with specific AI paradigms, such as Symbolic, Statistical, or both.
Controlling Technologies primarily focus on access and egress control in asset protection. Access control systems, sensors, credentials, and management systems fall under the Symbolic and Statistical AI Paradigms. Biometric systems, often incorporated into access control, align with the Statistical Paradigm. Vehicle and dispensable barrier mechanisms, as well as turnstile hardware, are classified under the Symbolic Paradigm. Currently, there are no Controlling technologies within the Sub-symbolic AI Paradigm.
Response Technologies involve neutralizing, containing, or mitigating security events. While human-guard force intervention remains a significant aspect of the response, technology has also become instrumental. Communication systems, dispensable barriers, vehicle barriers, and weaponry systems are examples of response technologies. These technologies fall within the Symbolic and Statistical AI Paradigms. Communications system devices and hardware align with the Symbolic Paradigm, while robotic system automation and management functions span Symbolic and Statistical Paradigms. Drone capture systems align with the Statistical Paradigm. Weapon systems encompass Symbolic sensors and Statistical analytics, while weapons management systems align with Symbolic and Statistical Paradigms.
Benefits and Opportunities
The development of Artificial Intelligence (AI) in security technologies holds great potential for various tangible benefits. One of the key advantages lies in the economic realm, where AI adoption can lead to increased productivity and cost reduction through the enhancement of specific AI tasks. However, the most significant benefit to humanity will be using response technologies like drones and robotics, which can remove humans from potentially dangerous situations.
As AI continues to evolve, numerous opportunities for its application in security technologies are expected to arise. These opportunities span different areas: observation, detection, control, response, and integrated security technologies.
1. AI Testing and Standardisation
In the realm of AI in security technologies, there are significant opportunities for standardization that can bring numerous benefits. One such opportunity lies in developing international AI standards, which can provide a common framework for designing and implementing AI systems across different countries and regions. Establishing these standards makes it easier to ensure interoperability and compatibility between systems, equipment, and devices.
Another area where standardization can play a crucial role is in the design and development of common connectivity protocols. Having uniform protocols that are widely adopted allows for seamless communication and integration between various platforms, equipment, and devices. This, in turn, reduces connectivity issues and facilitates data exchange, enabling greater intelligent interpretation and analysis.
Furthermore, the development and standardization of testing mechanisms are essential for driving accuracy and reliability in AI-based security technologies. By establishing standardized testing procedures, security managers can have greater confidence in the performance of systems under different operational conditions. Manufacturers and vendors can provide assurances regarding the proficiency levels of their systems, which helps security professionals make informed decisions about the suitability and effectiveness of AI technologies for their specific needs.
2. Technical Improvements
Regarding AI in security technologies, there is a constant drive for increased accuracy and reliability, especially in live operating environments and dynamic conditions. Achieving higher levels of accuracy and reliability is crucial for ensuring effective security measures. Alongside this, there are several areas where technical improvements can be pursued to enhance the capabilities of AI-based security technologies.
One area of focus for technical improvements is safety. It is essential to develop systems prioritizing safety measures to protect assets and individuals. Additionally, improvements in AI systems’ quality, resolution, and processing capabilities contribute to better overall performance and effectiveness. These advancements allow for more precise and detailed analysis, enabling security professionals to make informed decisions and take appropriate actions.
Reducing signal noise is another technical challenge that needs to be addressed. By minimizing unwanted or irrelevant data in the signals captured by security devices, AI algorithms can focus on the most critical information, improving the accuracy of threat detection and identification.
In terms of technical opportunities, the development of novel detection devices is an area with significant potential. Creating innovative sensors and devices can expand the range of threats that can be detected and provide more comprehensive security coverage. Moreover, the availability of diverse and high-quality source datasets plays a vital role in training AI models and improving their performance. Continued efforts in collecting and curating datasets relevant to security scenarios can enhance the accuracy and reliability of AI systems.
Increased mobility for sensors and devices is another aspect that can lead to technical improvements. By enabling sensors and devices to be more mobile and adaptable, security systems can be deployed in various environments and respond to changing threats effectively.
Lastly, observation technologies require advancements in object recognition and classification accuracy. Accurately identifying and classifying objects is crucial for effective surveillance and threat detection. Additionally, developing robust data management systems capable of handling large volumes of information produced by observation technologies is essential. These systems facilitate efficient data storage, processing, and retrieval, enabling effective analysis and decision-making.
3. Integration and Aggregation of Inputs
In the realm of security technologies, there is a growing recognition of the benefits derived from increased automation and greater integration of internal and external systems. By integrating various systems, such as building management and security systems, security technology can operate more cohesively and efficiently. This integration enables the aggregation of multi-sensor data, which, when combined with applied analytics, has the potential to significantly enhance security decision-making.
The use of advanced analytics on aggregated data from multiple sensors allows for a more comprehensive understanding of security situations. This distributed intelligence approach enables systems, facilities, and security teams to collaborate and exchange information, improving security responses. By leveraging the collective intelligence of interconnected systems, security measures can be more effective and adaptive.
To achieve this level of integration, security systems must transform a simple input/output architecture into a more sophisticated and coordinated decision-making architecture. This transformation involves establishing a seamless flow of information and decision-making processes between devices and the entire security field. It requires automation and management at multiple levels, such as Building Automation and Control Systems (BACS), to ensure a synchronized and efficient operation of security technologies.
Enhanced integration also offers improved usability and flexibility in accessing and controlling security systems and devices. For example, cross-platform control allows for seamless interaction and control over different systems or devices like drones and robotics during operations. This flexibility enables security personnel to leverage the strengths of various technologies and adapt their responses based on the specific requirements of each situation.
4. Analytics
Security technologies benefit greatly from the advancements in analytics and predictive analytics. These technologies offer significant opportunities for improving security measures, particularly in pattern recognition and anomaly detection. Developing novel analytical methodologies will be crucial to fully harness these opportunities.
Analytics play a vital role in supporting decision-making within security technologies. By analyzing vast amounts of data, analytics can identify patterns, trends, and anomalies that might go unnoticed through manual monitoring. This allows security practitioners to better understand their environment and make informed decisions based on real-time information. In dynamic environments, where conditions can rapidly change, analytics provides essential context for effective decision-making.
Furthermore, analytics can contribute to security technologies’ distributed intelligence and response systems. Analytics can provide dynamic assessments of security situations by continuously monitoring and assessing data. This enables security systems and teams to respond swiftly and appropriately to emerging threats or incidents. With analytics integration into security technologies, decision-making becomes more proactive and responsive, enhancing overall security effectiveness.
In conjunction with analytics, the concept of “ranked options” for response can be a valuable decision-making tool. These tools help security practitioners evaluate and prioritize different response options based on their probabilities of achieving desired outcomes. By considering multiple response scenarios and their associated probabilities, security practitioners can make compliant decisions that optimize the effectiveness of their actions.
Moreover, the collaboration between humans and machines, known as Human-Machine Teaming, can revolutionize critical decision-making in security operations. AI-generated options for response, based on advanced analytics and machine learning algorithms, can augment human operators’ decision-making processes. These AI-generated options can provide additional insights, alternative perspectives, and potential courses of action, thereby enhancing the overall decision-making capabilities of security practitioners.
5. Human-Machine Teaming
Human-Machine Teaming (HMT) represents a paradigm emphasizing the collaborative development and integration of humans and machines to achieve specific goals and capabilities. Unlike fully autonomous systems that replace human involvement, HMT seeks to assist and augment human activities rather than replace them. This collaborative approach has significant potential across various security technologies, focusing on Response technologies like drones and robotics.
Maintaining human control over systems and devices is essential in the context of Response technologies, where trust and safety are critical considerations. HMT provides an opportunity to combine the unique strengths of humans and machines, leveraging human judgment, intuition, and decision-making capabilities alongside machines’ precision, efficiency, and automation. By working collaboratively, humans and machines can enhance security operations while upholding the importance of human oversight.
Integrated security management systems are a prime example of where HMT can offer substantial improvements and benefits for security operators. HMT can facilitate seamless coordination and communication between human operators and intelligent machines by integrating various security technologies and systems, such as surveillance, access control, and incident response. This integration ensures that critical decisions remain under human control, preventing potential compromises to security and safety.
Through HMT, security operators can leverage the capabilities of machines, such as drones and robotics, to perform potentially dangerous or physically challenging tasks. Machines can assist in surveillance, reconnaissance, and response activities, while human operators retain the authority to make critical decisions based on their expertise and situational awareness. This collaborative approach enhances operational efficiency, response times, and overall effectiveness while maintaining the necessary human judgment in complex security scenarios.
By embracing HMT within integrated security management systems, security operators can benefit from improved situational awareness, increased operational capabilities, and enhanced decision-making support. The collaboration between humans and machines can optimize security operations without compromising critical decisions or compromising the safety and security of individuals. HMT represents a promising avenue for advancing security technologies, ensuring human-centric control, and achieving optimal security outcomes.