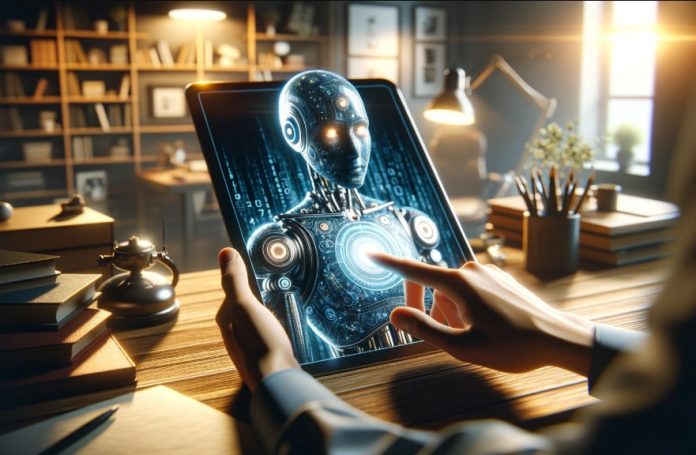
The evolution of AI technologies has led to the introduction of new concepts and applications that shape the future of various industries. Generative AI and Agentic AI are gaining significant attention, mainly due to their potential to revolutionize content creation, automation, and business workflows.
While generative AI has dominated the conversation in recent years, there’s a rising shift towards a more autonomous, goal-driven approach known as Agentic AI. This shift could redefine the way AI systems are utilized across various sectors. Let’s delve into Agentic AI, how it differs from traditional generative AI, and why it’s gaining so much attention.
What is Agentic AI?
Agentic AI, often called Autonomous AI, represents a step beyond generative capabilities. While generative AI creates content, Agentic AI is designed to perform autonomous tasks to achieve a specific goal or business outcome. It goes beyond simply generating text or images by integrating tools and external data sources to accomplish complex workflows independently, without human intervention.
How Agentic AI Works:
- Autonomous Action: Agentic AI can autonomously integrate and interact with various external systems (like APIs, databases, news sources, etc.) to achieve a set goal. This could be anything from analyzing stock market data to automating customer service responses or solving logistical problems.
- Tool Integration: Agentic AI applications are typically designed to connect to external tools, like web scraping tools, APIs, or even databases, to gather and process the most relevant and up-to-date information needed to accomplish their tasks. For example, an agentic AI might access financial data to make stock-buying recommendations or gather customer feedback to refine a marketing campaign.
- Complex Workflow Execution: One of the most powerful features of Agentic AI is its ability to execute complex workflows that involve multiple stages of data collection, analysis, and decision-making. These workflows are often dynamic, meaning the AI agents may modify their actions or decisions as the process unfolds.
- Self-Improvement: Unlike generative AI, which focuses on producing content, Agentic AI is capable of self-learning and fine-tuning its behavior over time. As it gathers more information and completes tasks, it can adapt and improve its performance, leading to higher efficiency and more accurate results.
What Makes Agentic AI Autonomous?
Agentic AI takes generative AI a step further by incorporating autonomy. Here’s the key difference:
Generative AI creates content, like articles, poems, or summaries, based on predefined prompts.
On the other hand, Agentic AI performs actions autonomously to complete specific tasks, often involving multiple steps and interacting with external systems or tools to achieve a business or operational outcome.
For example, in a financial advisor AI system, an agentic AI could autonomously gather data from various financial tools, analyze market trends, and provide recommendations on stock investments—all without human intervention. This is a far more dynamic process than merely generating a financial report from a prompt.
At its core, agentic AI is designed to work towards a specific goal. To achieve this, the system interacts with several external tools, collects and analyzes data from various sources, and follows a structured workflow. The beauty of this approach lies in its flexibility: the system can adapt its process depending on the information it retrieves, optimize its performance, and even fine-tune itself to enhance its task completion.
For example, imagine an agentic AI tasked with recommending stocks to buy. It would autonomously:
- Gather the latest stock data from sources like YT Finance.
- Search for relevant news articles or sentiment analysis regarding those stocks.
- Analyze historical stock performance.
- Finally, provide a recommendation on which stock to purchase.
Each step is executed independently by different AI agents, all working in parallel to achieve the overall goal.
The Frameworks Powering Agentic AI
Several new frameworks are emerging to facilitate the development of agentic AI applications. These frameworks allow developers to create complex workflows, integrate various external tools, and deploy autonomous AI agents efficiently. Here are some of the most notable frameworks:
- Langchain and Langflow: These frameworks allow for the creation of autonomous workflows, enabling the integration of LLMs with external tools. For example, with Langflow, developers can create agentic AI systems using a no-code or low-code approach, making it accessible for seasoned developers and newcomers.
- FData: This framework aims to create agentic AI agents for specific tasks such as legal analysis, financial advising, etc. It allows for integrating multiple tools and LLMs to achieve complex workflows.
- Microsoft Autogen: A more recent addition to the AI landscape, Autogen is an open-source framework that enables the development of agentic AI systems with powerful automation and tool integration capabilities.
These frameworks empower developers to create more sophisticated and goal-oriented AI systems, ushering in a new era of autonomous technology.
Applications of Agentic AI:
- Automated Financial Advisors: Agentic AI can interact with real-time market data, financial APIs, and news sources to offer autonomous financial advice, such as stock recommendations or risk analysis, without human oversight.
- Customer Service Automation: In complex customer service workflows, Agentic AI agents can autonomously interact with various internal systems to handle tasks like troubleshooting, ticket escalation, and customer feedback analysis.
- Supply Chain Management: Agentic AI can optimize supply chain logistics by autonomously interacting with inventory management systems, demand forecasting tools, and shipping carriers, all while learning and adapting based on operational data.
The Future of Agentic AI
As businesses increasingly recognize the potential of agentic AI, its use cases are becoming more apparent. From personalized financial advisors to healthcare assistants who can autonomously monitor patient conditions, agentic AI is poised to revolutionize various industries. The key advantages include:
- Efficiency: With autonomous systems, tasks can be completed faster and without human oversight.
- Adaptability: Agentic AI can learn and fine-tune its processes based on real-time data, improving its performance over time.
- Scalability: Complex workflows involving multiple tasks and external tools can be scaled effortlessly.
Companies that embrace agentic AI will streamline their operations and provide more sophisticated and personalized services to their customers.
Conclusion
Agentic AI represents the future of artificial intelligence—autonomous, goal-oriented, and capable of handling complex tasks without human intervention. While generative AI has significantly impacted content creation, agentic AI takes this further by executing workflows and making intelligent decisions. With powerful new frameworks making agentic AI more accessible, it’s only a matter of time before these autonomous systems become integral to businesses and everyday life. The rise of agentic AI is an exciting development, and as we move forward, its impact will undoubtedly shape the next generation of AI-powered technologies.