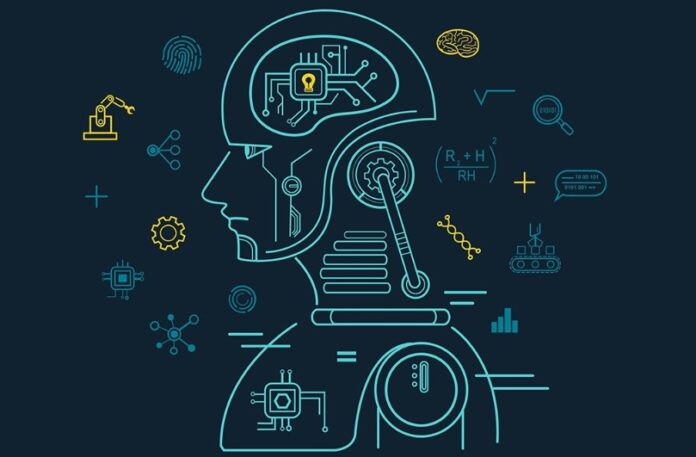
In recent years, deep learning has rapidly evolved from a niche field of research to a dominant force in artificial intelligence (AI). As companies strive to maintain a competitive edge, deep learning has emerged as a key tool for solving complex problems and enhancing user experiences. But why is deep learning becoming so popular?
Let’s explore the reasons behind this trend and understand its growing impact.
1. Exponential Growth in Data
One of the primary reasons for the rise of deep learning is the explosion of data. As more and more people engage with digital platforms—social media, e-commerce, or entertainment—an enormous amount of data is generated. Platforms like Facebook, Instagram, YouTube, and even smartphones contribute terabytes of data every second. This vast data pool is invaluable for training deep learning models, enabling them to perform better over time.
In the past, machine learning algorithms could not handle such large datasets effectively. Their performance would plateau after a certain point, and they would be unable to leverage the increasing volume of data. However, deep learning models, particularly deep neural networks, benefit from more data, allowing them to improve accuracy and efficiency continuously.
2. Improved Performance with Larger Datasets
Deep learning shines when it comes to handling large amounts of data. Unlike traditional machine learning models, which tend to degrade in performance as data volume increases, deep learning models continue to improve. This is evident from the performance metrics, where deep learning models consistently outperform older algorithms as data grows. The ability of deep learning models to scale and adapt to large datasets has been a game-changer, especially in fields like image recognition, speech processing, and natural language understanding.
3. Advancements in Affordable Hardware
The accessibility of powerful hardware has played a significant role in the popularity of deep learning. The cost of hardware required to train deep learning models, particularly Graphics Processing Units (GPUs), has drastically decreased over time. Companies like NVIDIA have been at the forefront of this revolution, creating GPUs that are not only more affordable but also optimized for deep learning tasks.
Additionally, the advent of cloud computing has made it easier for organizations to access high-performance GPUs without costly physical infrastructure. Cloud providers offer on-demand GPU services, which means businesses and researchers can now scale their operations without breaking the bank. These advancements have allowed smaller organizations to dive into deep learning, leveling the playing field.
4. Simplified Feature Extraction in Deep Learning
In traditional machine learning, feature engineering is a critical step that involves manually extracting relevant features from raw data. This process can be time-consuming and often requires domain expertise. However, feature extraction is integrated directly into the model training process in deep learning.
Deep learning models, such as convolutional neural networks (CNNs), can automatically learn and extract the features from data without manual intervention. This makes deep learning more efficient and less reliant on human expertise, making it easier for developers to apply these models to a wide variety of tasks.
5. Ability to Solve Complex Problems
Deep learning has proven especially effective in solving complex problems that were once thought to be beyond the reach of AI. Thanks to deep learning techniques, tasks such as image classification, object detection, and speech recognition have all seen remarkable advancements.
For instance, deep learning models can now identify objects in images with remarkable accuracy, recognize speech with near-human precision, and even understand and generate human language. These breakthroughs have led to the proliferation of applications in fields like healthcare, autonomous driving, and entertainment, further driving the popularity of deep learning.
6. The Convergence of Multiple Factors
The rise of deep learning can be attributed to the confluence of several factors:
- Data: The unprecedented growth of data has provided the fuel for deep learning models.
- Hardware: Cheaper, more powerful hardware has made deep learning accessible to a broader audience.
- Algorithms: Advances in neural network architectures have enabled models to solve more complex problems.
- Cloud Computing: Cloud-based GPU services allow even small businesses and researchers to use deep learning technologies.
These factors have created an environment where deep learning can flourish, enabling businesses to solve problems more efficiently and develop innovative solutions.
Conclusion
Deep learning is becoming more popular because it enables more accurate models and solves problems that were previously considered too complex. With the increasing availability of data, affordable hardware, and sophisticated algorithms, deep learning has become an indispensable tool for companies and researchers. As these trends continue, deep learning will likely play an even more prominent role in shaping the future of AI and technology.