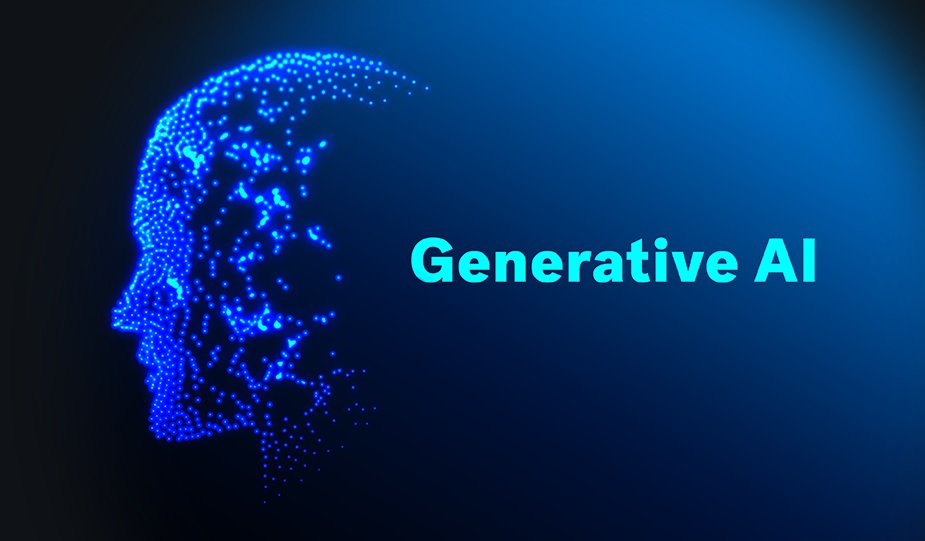
Generative AI has created quite a buzz in the tech industry over the last couple of years, particularly in data analytics. The advancements have been remarkable from large language models (LLMs) by tech giants like Google, Meta, OpenAI, Microsoft, and Anthropic to multimodal models capable of text, image, and video generation. However, amidst the excitement, the question arises: when is generative AI genuinely compelling, and when is it not? This article explores this question in depth.
The Strengths of Generative AI
Generative AI shines in two primary areas: content generation and conversational user interfaces (CUIs).
- Content Generation: Generative AI excels in creating text, images, videos, and even synthetic data. Whether generating marketing content, designing visuals, or crafting personalized messages, these capabilities have revolutionized the media, advertising, and entertainment industries.
- Conversational User Interfaces: Applications like virtual assistants, chatbots, and digital workers thrive on generative AI. These tools can simulate human-like conversations, automate support processes, and provide a seamless user experience, making them indispensable for businesses aiming to enhance customer engagement and satisfaction.
The key to success in these areas is generative AI’s ability to process vast amounts of data and generate coherent, creative outputs. This makes it a game-changer for industries where automation and personalization are crucial.
Limitations of Generative AI
Despite its strengths, generative AI is not universally effective. Here are the domains where it faces challenges:
- Prediction and Forecasting: Generative AI struggles with tasks like risk prediction, customer churn forecasting, or sales demand estimation. Traditional machine learning (ML) and deep learning (DL) models, trained on specific datasets, often outperform generative AI in these areas due to their higher accuracy and reliability.
- Decision Intelligence: When it comes to complex decision-making systems that require precise data analysis, generative AI falls short. Established ML algorithms provide better results by leveraging domain-specific data.
- Segmentation and Classification: While generative AI can handle these tasks, the results are often mediocre compared to specialized ML techniques. Accurate segmentation and classification are critical in healthcare and finance, where precision is non-negotiable.
- Recommendation Systems: Like those used in e-commerce or streaming platforms, recommendation engines rely on specific patterns and preferences. Generative AI lacks the robustness and efficiency of traditional ML techniques in delivering high-accuracy recommendations.
Why Generative AI Isn’t Always the Answer
The widespread hype around generative AI has led to its application in areas where it is not a good fit. Here’s why this can backfire:
- Increased Complexity and Risk of Failure: Forcing generative AI into unsuitable use cases can increase project complexity and the likelihood of failure. The hype often blinds decision-makers to more appropriate solutions.
- Overlooking Established Techniques: Generative AI’s buzz often overshadows traditional AI methods like ML and DL, which are well-suited for many business challenges. Ignoring these tried-and-tested techniques can result in suboptimal outcomes.
- Lack of Versatility in Generative AI Models: Generative AI models are excellent at creating new content but lack the adaptability for analytical or operational tasks.
Striking a Balance: Where Generative AI Fits
To maximize effectiveness, organizations must adopt a balanced approach:
- Identify the Right Use Case: Before deploying generative AI, evaluate whether the problem requires content generation or conversational capabilities. If not, traditional techniques might be more suitable.
- Combine AI Techniques: Combining generative AI with ML or DL can yield better results for complex projects. For instance, using ML for data segmentation and generative AI for personalized content can create a robust solution.
- Invest in a Strong Foundation: While generative AI is trending, professionals should not overlook the importance of understanding ML and DL fundamentals. These skills remain relevant and essential for solving diverse use cases.
The Future of Generative AI
Generative AI’s popularity is fueled by its ability to address high-demand tasks like chatbots and content creation. However, the hype may eventually settle as the technology matures and becomes commonplace. When that happens, the focus will likely shift back to the broader AI ecosystem, emphasizing traditional methods for analytical and operational tasks.
To stay ahead, organizations and individuals must maintain a well-rounded skill set. While mastering generative AI is valuable, a comprehensive understanding of all AI techniques is the key to long-term success.
Conclusion
Generative AI is a powerful tool with undeniable strengths in content creation and conversational interfaces. However, its effectiveness is limited in prediction, classification, or decision-making tasks. By recognizing its capabilities and limitations, businesses can make informed decisions about when and how to use generative AI, ensuring optimal results while avoiding unnecessary complexity.
The AI landscape is evolving rapidly, and staying adaptable is crucial. Combining generative AI with established techniques will enable businesses to harness the full potential of AI, driving innovation and delivering value to customers.