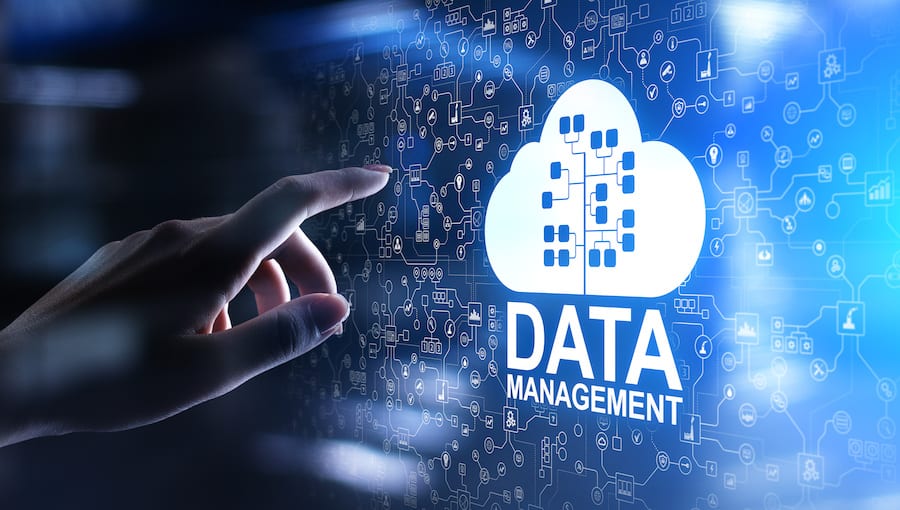
Choosing the right data management tool is essential for enterprises seeking to harness the power of data, improve efficiency, ensure data quality, maintain security and compliance, and gain a competitive edge in today’s data-centric business environment. This strategic decision can significantly impact an organization’s success in the digital age.
In today’s business landscape, data is often likened to the “new oil” due to its immense value in providing insights, identifying trends, and supporting informed decision-making. The appropriate data management tool ensures that data remains accurate, accessible, and well-organized, enabling enterprises to base their decisions on reliable information.
Data management tools also streamline data-related processes, reducing the manual effort required for tasks such as data integration, cleansing, and transformation. This, in turn, enhances efficiency and productivity within an organization, freeing up employees to focus more on strategic activities. Additionally, these tools facilitate the collection and analysis of customer data, empowering organizations to tailor their offerings and services to individual needs.
Key Features of Data Management Tools
- Data Integration: The ability to connect to and extract data from various sources, including on-premises databases, cloud-based applications, and social media.
- Data Transformation: The capacity to clean, format, and transform data to prepare it for analysis.
- Data Quality Management: The capability to identify and rectify errors in data.
- Data Governance: The ability to establish and enforce policies for data access, use, and security.
- Data Visualization: The capacity to create charts, graphs, and other visualizations to explore and comprehend data.
- Data Analytics: The ability to perform statistical and analytical operations on data to extract insights.
This article presents a list of the top 15 data management tools that enterprises should consider to enhance their strategic decision-making capabilities and thrive in the digital age.
1. Profisee
Profisee is a comprehensive data management tool designed to empower organizations in mastering their data assets. Its standout features include robust Master Data Management (MDM) capabilities that enable the creation of a centralized hub for critical data like customer, product, and supplier information. Profisee also offers robust data quality management tools for profiling, cleansing, and standardizing data, ensuring data accuracy and consistency. The platform facilitates data governance through policy enforcement and compliance with data regulations. Moreover, Profisee excels in data integration, supporting connections to diverse data sources, both on-premises and in the cloud. Additionally, it streamlines data workflows and fosters collaboration within teams.
Pros
Its comprehensive MDM solution offers a holistic approach to data management, covering data quality, governance, and integration. Users often praise its user-friendly interface, making it accessible across an organization. Scalability is another strong point, allowing Profisee to grow with an organization’s evolving data management needs. Its data quality tools are robust and effective in enhancing data accuracy and reliability. Moreover, the platform’s customization options ensure that it can be tailored to align with specific business processes and requirements.
Cons
The cost of Profisee may be prohibitive for smaller businesses, potentially limiting its accessibility. While user-friendly, there might still be a learning curve, especially for those new to MDM and data management concepts. Integrating Profisee with existing IT systems could be challenging, depending on an organization’s infrastructure. These factors should be weighed against the potential benefits when considering adoption.
2. Looker
Looker is a powerful business intelligence (BI) and data analytics platform that empowers organizations to explore and analyze their data for better decision-making. Its core features include a user-friendly interface that allows users to create and share interactive data visualizations and reports without requiring extensive technical skills. Looker offers a centralized data model, providing a single source of truth for data across an organization. It supports data integration from various sources, both cloud-based and on-premises, making it versatile for different data setups. Looker’s robust data modeling and transformation capabilities enable users to manipulate and structure data to suit their specific analysis needs. It also supports collaboration through data sharing and scheduled report distribution.
Pros
Looker offers numerous advantages. Its ease of use and self-service capabilities allow non-technical users to create and explore data visualizations, reducing dependence on IT departments. The platform promotes data-driven decision-making by providing real-time access to data, fostering a culture of data-driven insights. Looker’s centralized data model ensures data consistency and accuracy, crucial for organizations seeking reliable insights. The tool’s ability to integrate with various data sources, including cloud data warehouses, positions it well for modern data ecosystems. Looker’s robust community and marketplace provide a wide range of pre-built templates and extensions, saving time in report creation.
Cons
Looker’s pricing can be relatively high, making it less accessible for smaller organizations. The platform’s extensive capabilities may have a learning curve for new users, particularly those unfamiliar with BI and data analysis concepts. While Looker supports integration with various data sources, setting up these integrations can be complex, depending on an organization’s existing infrastructure. Some users may find that the platform’s customization options, while powerful, require technical expertise to implement fully.
3. Panoply
Panoply is a cloud-based data warehouse and management platform designed to simplify the process of data integration and analytics for organizations. One of its primary features is its ability to automatically collect, transform, and store data from various sources, including databases, SaaS applications, and cloud services. Panoply uses machine learning to automate data modeling and schema creation, allowing users to query and analyze data without the need for extensive coding or data engineering. It supports real-time data syncing, ensuring that the data is always up-to-date. Panoply integrates with popular business intelligence (BI) tools, making it easy to visualize and analyze data.
Pros
Panoply offers several advantages. Its automation features save time and reduce the complexity of data integration and transformation, particularly valuable for organizations lacking dedicated data engineering resources. The platform is scalable and can handle large volumes of data, making it suitable for businesses of all sizes. Panoply’s integration with various data sources, including cloud data warehouses, simplifies the process of centralizing and managing data. Its compatibility with BI tools like Tableau and Looker allows for seamless data analysis and reporting.
Cons
While Panoply is user-friendly, there can still be a learning curve for users who are new to data warehousing and analytics. Some users have reported limitations in terms of data transformation capabilities, particularly for complex data manipulation tasks. Pricing can be a concern for smaller organizations, as the cost may increase as data volumes grow. Additionally, while Panoply supports a wide range of data sources, there may be specific integrations that require custom development.
4. Stitch Data
Stitch Data is a cloud-based data integration platform that simplifies the process of extracting, transforming, and loading (ETL) data from various sources into data warehouses. One of its key features is its support for over 100 data sources, including popular databases, SaaS applications, and cloud services. Stitch Data offers a user-friendly interface for configuring data pipelines, allowing users to set up data integration tasks without extensive coding or data engineering expertise. It provides real-time data replication, ensuring that the data in your warehouse is always up-to-date. Stitch Data also supports data transformation and enrichment, enabling users to prepare data for analysis.
Pros
Stitch Data offers several advantages. Its extensive list of supported data sources makes it a versatile choice for businesses with diverse data ecosystems. The platform’s ease of use and no-code/low-code approach to ETL simplifies data integration for both technical and non-technical users. Real-time data replication ensures that organizations have access to the most current data, crucial for making informed decisions. Stitch Data is scalable, allowing businesses to handle growing data volumes. It also provides monitoring and alerting features to keep track of data pipeline performance.
Cons
While Stitch Data offers a wide range of data sources, some less common sources may not be supported, requiring custom solutions. The platform’s data transformation capabilities, while useful for many tasks, may not be as robust as some dedicated data transformation tools, potentially limiting more complex data manipulation. Pricing can be a concern for smaller organizations, as costs may increase with the volume of data processed. Additionally, some users may find that the platform’s documentation could be more extensive to support their integration needs.
5. Microsoft Power BI
Microsoft Power BI is a powerful business intelligence (BI) platform that enables organizations to visualize and analyze their data for informed decision-making. Its features include data visualization tools that allow users to create interactive and customized reports and dashboards. Power BI supports data integration from a wide range of sources, including databases, cloud services, and on-premises data. It offers advanced data modeling capabilities for transforming and structuring data for analysis. The platform includes natural language querying, enabling users to ask questions and receive answers from their data. Power BI also supports real-time data streaming and collaboration features, making it a comprehensive BI solution.
Pros
Power BI offers several advantages. It has a user-friendly interface that caters to both technical and non-technical users, reducing the reliance on IT departments for report creation. The platform’s integration with Microsoft’s ecosystem, including Azure and Office 365, provides seamless connectivity and collaboration. Power BI’s robust data visualization options make it easy to create compelling and interactive dashboards. It offers both cloud and on-premises deployment options, accommodating various organizational preferences. Regular updates and a thriving community mean that users have access to a wealth of resources and features.
Cons
The cost of Power BI can be a drawback for smaller organizations, as advanced features may require premium licensing. While user-friendly, there can still be a learning curve, especially for users new to BI and data analysis concepts. Complex data modeling and transformations may require a deeper understanding of Power Query and DAX, which could be challenging for some users. Additionally, while Power BI supports a wide range of data sources, there may be specific integrations that require custom development.
6. Google Cloud Platform
Google Cloud Platform (GCP) offers a robust suite of tools and services for data management and analytics. It provides a secure and scalable infrastructure for storing and processing data, catering to organizations of all sizes. Within GCP, you’ll find BigQuery, a fully managed, serverless data warehouse enabling users to analyze massive datasets through SQL queries. Additionally, it offers Cloud Storage for object storage, Cloud Datastore for NoSQL data, and Cloud Spanner for globally distributed databases. GCP simplifies data integration with tools like Dataflow and Dataprep and supports machine learning and AI via services like AI Platform and TensorFlow, enabling advanced data analytics and predictive modeling.
Pros
There are several advantages to using Google Cloud Platform for data management. GCP’s scalability ensures organizations can handle growing data volumes without infrastructure limitations. Its global network infrastructure provides low-latency data access and services worldwide. Google’s expertise in data processing and analytics shines through services like BigQuery, offering high-speed querying and seamless integration with other GCP tools. The platform’s robust security features, including encryption, identity and access management, and compliance certifications, make it a reliable choice for handling sensitive data. GCP’s data analytics and machine learning capabilities empower organizations to extract valuable insights from their data.
Cons
GCP’s pricing model can be intricate, and costs can escalate quickly, particularly for resource-intensive workloads. Users may need to closely monitor and optimize their usage to manage expenses effectively. While GCP offers a wide range of services, selecting and configuring the right combination of tools may pose a challenge for users new to cloud computing. Data integration and transformation processes may require some level of technical expertise. Additionally, as with any cloud provider, organizations should consider data governance and compliance when storing and processing data on GCP.
7. Amazon Web Services (AWS)
Amazon Web Services (AWS) provides a comprehensive array of services and tools for data management, storage, and analytics. AWS includes Amazon S3 for scalable and cost-effective object storage, suitable for data storage and archiving. Amazon RDS offers managed relational databases, while Amazon Redshift is a fully managed data warehouse service designed for analytics. AWS Glue simplifies data integration and ETL (Extract, Transform, Load) processes, facilitating data preparation for analysis. AWS also offers various analytics services, including Amazon Athena for ad-hoc querying, Amazon EMR for big data processing, and Amazon QuickSight for data visualization and business intelligence. AWS empowers organizations to build data lakes, implement real-time analytics, and harness machine learning for data-driven insights.
Pros
Using AWS for data management comes with several advantages. AWS boasts a global network of data centers, ensuring low-latency access to data and services worldwide. Scalability enables organizations to handle data growth without infrastructure constraints. AWS employs a pay-as-you-go pricing model, allowing users to pay only for the resources they consume, potentially resulting in cost savings. The platform’s security features, including encryption, identity and access management, and compliance certifications, guarantee data remains secure and compliant. AWS also offers a rich ecosystem of third-party integrations and a strong developer community, providing flexibility and support for various use cases.
Cons
AWS’s pricing can become complex, and organizations may need to carefully manage costs, especially for resource-intensive workloads. New AWS users may find the array of services and configuration options overwhelming, necessitating a learning curve. Data integration and ETL processes may still require technical expertise, and organizations should be cautious about potential data egress costs when moving data out of AWS. Additionally, while AWS provides robust security measures, organizations are responsible for configuring and managing security settings correctly.
8. Informatica
Informatica stands as a leading data management platform, offering a comprehensive suite of tools and services for organizations to manage, integrate, and analyze their data. It encompasses data integration, data quality, data governance, and cloud data management. One of its flagship products, Informatica PowerCenter, empowers users to connect to various data sources, transform data, and load it into target systems with robust data integration capabilities. Informatica Data Quality ensures data accuracy and consistency through profiling, cleansing, and standardization. The platform supports data governance with features for data cataloging, data lineage, and policy enforcement. Informatica also offers cloud-based solutions like Informatica Cloud for seamless integration with cloud applications and data warehousing.
Pros
Informatica offers numerous advantages. Its versatility enables organizations to manage data across diverse environments, including on-premises, cloud, and hybrid setups. The platform’s user-friendly interface caters to both technical and non-technical users, reducing the reliance on IT departments for data-related tasks. With robust data integration capabilities, Informatica handles large data volumes effortlessly, ensuring data availability when needed. The data quality features contribute to improved data accuracy and reliability, facilitating better decision-making. Additionally, Informatica’s support for cloud data management aligns well with modern data ecosystems.
Cons
Informatica’s pricing model can be complex, with costs varying based on specific components and usage scale. Smaller organizations might find it challenging to justify the investment due to costs. While user-friendly, there may still be a learning curve, especially for those new to data integration and management concepts. Integrating Informatica with an organization’s existing IT infrastructure may require effort and expertise. Additionally, implementing data governance practices within an organization, although supported comprehensively by Informatica, can be a complex and time-consuming process.
9. IBM InfoSphere
IBM InfoSphere serves as a comprehensive data management platform designed to help organizations effectively manage, integrate, and govern their data assets. It offers a range of features to support various data-related tasks. InfoSphere Information Server, a core component, equips users with robust data integration capabilities, enabling them to connect to diverse data sources, transform data, and load it into target systems. The platform includes data quality tools for profiling, cleansing, and standardizing data, ensuring data accuracy and consistency. InfoSphere Information Governance Catalog helps organizations catalog and manage their data assets, providing visibility into data lineage and governance. Additionally, InfoSphere offers data replication, data masking, and data archiving features to support various data management needs.
Pros
IBM InfoSphere offers several advantages. Its scalability caters to organizations of all sizes, from small businesses to large enterprises. The platform’s robust data integration and data quality capabilities ensure reliable and accurate data, essential for informed decision-making. InfoSphere Information Governance Catalog offers a centralized repository for managing data assets, enhancing data governance and compliance efforts. IBM’s reputation for security and compliance is reflected in InfoSphere’s features, making it a trusted choice for handling sensitive data. The platform’s support for various data sources and formats ensures flexibility in data management.
Cons
IBM InfoSphere can be relatively complex to implement and configure, demanding significant time and resources. The pricing structure may be on the higher side, potentially limiting accessibility for smaller organizations with budget constraints. While powerful, InfoSphere may present a steeper learning curve for users new to data integration and governance concepts. Integrating InfoSphere with existing IT systems and workflows may require careful planning and technical expertise. Additionally, like many comprehensive data management solutions, it may take time to fully realize the benefits of InfoSphere’s implementation.
10. Talend
Talend stands as a robust data integration and transformation platform designed to assist organizations in managing and processing their data effectively. It offers a comprehensive suite of features for data integration, data quality, data governance, and data analytics. Talend provides a user-friendly, drag-and-drop interface for designing data integration workflows, ensuring accessibility for both technical and non-technical users. It supports connectivity to a wide range of data sources, including databases, cloud services, and on-premises systems. Talend’s data quality tools enable users to profile, cleanse, and standardize data, ensuring data accuracy and consistency. The platform includes data governance features for managing data lineage, data cataloging, and compliance with data regulations. Additionally, Talend supports big data and cloud data integration, making it suitable for modern data ecosystems.
Pros
Talend offers several advantages. Its ease of use and visual interface reduce the learning curve, allowing organizations to swiftly set up data integration processes. The platform’s open-source version, Talend Open Studio, provides a cost-effective option for smaller organizations or individuals. Talend’s scalability accommodates both small and large data volumes, catering to businesses of varying sizes. The platform’s strong community support and extensive library of pre-built connectors and components save time and effort in integration tasks. Talend’s flexibility and support for various deployment options, including cloud and on-premises, provide versatility to organizations.
Cons
The pricing for Talend’s enterprise versions can be on the higher side, potentially limiting accessibility for smaller organizations with budget constraints. While Talend offers a user-friendly interface, more complex data integration and transformation tasks may still require technical expertise. Some users have reported performance variations depending on the complexity of data transformations and the volume of data processed. Additionally, while Talend provides data governance features, organizations should carefully plan and configure their governance processes to fully realize their benefits.
11. Matillion
Matillion serves as a data integration and transformation platform purpose-built for cloud data warehouses like Amazon Redshift, Google BigQuery, and Snowflake. It offers organizations a range of features to simplify and expedite data management processes. Matillion offers an intuitive, no-code/low-code user interface that enables users to design and schedule data workflows with ease. It supports data extraction from various sources, data transformation, and loading into cloud data warehouses. The platform includes connectors for popular data sources, simplifying integration with databases, SaaS applications, and cloud services. Matillion also offers transformation capabilities, including data enrichment, aggregation, and data cleansing, allowing users to build, schedule, and monitor data pipelines efficiently.
Pros
Matillion boasts several advantages. Its cloud-native design leverages the scalability and flexibility of cloud data warehouses, enabling organizations to handle substantial data volumes. The platform’s user-friendly interface caters to both technical and non-technical users, reducing the need for extensive coding or data engineering expertise. Matillion provides a library of pre-built data transformation components, speeding up development and saving time for users. Its integration with cloud data warehouses ensures real-time data processing and analytics. Matillion’s strong community and support ecosystem offer users a wealth of resources and expertise.
Cons
The pricing for Matillion can vary depending on the specific cloud data warehouse and the volume of data processed, potentially leading to unexpected costs for some organizations. While Matillion is user-friendly, some complex data transformation tasks may still require technical expertise. Organizations should carefully plan their data integration processes to ensure optimal performance and scalability. Additionally, Matillion’s focus on cloud data warehouses may not be suitable for organizations heavily reliant on on-premises data storage and processing.
12. Snowflake
Snowflake stands out as a cloud-based data warehousing platform known for its scalability and flexibility. It offers various features for data management and analytics. Snowflake’s architecture separates storage and compute, enabling users to scale each component independently. This allows organizations to store large amounts of data cost-effectively and allocate compute resources as needed for data processing and analytics. Snowflake supports multiple data formats and seamlessly integrates with popular business intelligence (BI) and analytics tools. It includes features for data sharing, replication, and security, making it suitable for diverse data needs. Additionally, Snowflake provides a SQL-based interface for querying and analyzing data.
Pros
Snowflake offers several benefits. Its cloud-native design ensures high scalability, enabling organizations to handle expanding data volumes without infrastructure limitations. The platform provides near-instant elasticity, allowing users to adjust compute resources as needed, which can lead to cost savings. Snowflake’s data sharing capabilities simplify secure data sharing with external partners. It boasts robust data security features, including encryption and access control, essential for safeguarding sensitive data. The integration with popular BI and analytics tools ensures a familiar and smooth user experience.
Cons
However, there are factors to consider. Snowflake’s pricing model can be complex, requiring careful management of compute and storage costs to avoid unexpected expenses. While Snowflake excels with structured data, handling semi-structured or unstructured data may involve additional data preparation. Users transitioning from traditional data warehousing systems may encounter a learning curve due to Snowflake’s unique architecture and SQL dialect. Some advanced analytics features may not be as comprehensive as those in specialized data analytics platforms, which may necessitate the use of additional tools alongside Snowflake.
13. Redshift Spectrum
Amazon Redshift Spectrum serves as an extension of Amazon Redshift, a cloud data warehousing service, allowing users to query data directly from Amazon S3, the cloud object storage service. It enables SQL queries on data stored in S3 without the need to load the data into Redshift. Key features of Redshift Spectrum include seamless integration with Amazon Redshift, providing a unified querying interface for both Redshift cluster data and S3 data. It supports various data formats, including Parquet, ORC, Avro, and JSON, offering flexibility for diverse data sources. Redshift Spectrum leverages massively parallel processing (MPP) for high-performance complex queries on large datasets, coupled with serverless and on-demand pricing.
Pros
Redshift Spectrum offers several advantages. It allows users to query and analyze vast data stored in Amazon S3, leveraging existing data lakes for analytics, reducing data movement and storage costs. Integration with Amazon Redshift enables the combination of data from the data warehouse and S3 for comprehensive analytics. Redshift Spectrum’s on-demand pricing model means organizations pay only for the queries they run, making it cost-effective for sporadic or unpredictable workloads. The platform is highly scalable, accommodating large datasets and complex queries.
Cons
While Redshift Spectrum provides a robust querying interface, complex and resource-intensive queries may require tuning for optimal performance. Query costs can accumulate, especially for organizations with extensive querying needs, necessitating monitoring and optimization. Redshift Spectrum’s integration with Redshift makes it most suitable for organizations already using or planning to use Redshift as their data warehousing solution. Users transitioning from traditional data warehousing systems may face a learning curve, as SQL queries may need adjustments for Redshift Spectrum’s architecture.
14. Databricks
Databricks serves as a unified analytics platform designed to simplify and expedite data management, analytics, and machine learning tasks. A standout feature is its support for Apache Spark, a robust open-source framework for big data processing and analytics. Databricks offers a collaborative workspace facilitating seamless collaboration among data engineers, data scientists, and analysts. It provides tools for data integration, transformation, and exploration, supporting various data sources, including data lakes and structured databases. The platform includes machine learning libraries and frameworks for AI model development and deployment. Databricks offers automated cluster management, allowing users to adjust resources for data processing and analytics.
Pros
Databricks offers several benefits. Its collaborative workspace fosters efficient cross-functional collaboration among data teams. Integration with Apache Spark ensures high-performance data processing and analytics, making it suitable for large datasets and complex tasks. Databricks simplifies data engineering with auto-scaling, automated job scheduling, and a no-code/low-code interface for data transformation. It supports advanced analytics and machine learning, serving as a comprehensive platform for organizations aiming to leverage data for insights and predictions. Databricks can be deployed both in the cloud and on-premises, providing flexibility in data management.
Cons
Databricks’ pricing model can be complex, with costs escalating based on resource usage, necessitating careful spending management. While user-friendly, the platform may entail a learning curve, particularly for users new to Apache Spark or machine learning concepts. Organizations with existing data infrastructure may encounter integration challenges when adopting Databricks. Customizing the platform may require coding and technical expertise, which could be a limitation for some users. Additionally, while Databricks offers a range of machine learning tools, organizations with highly specialized machine learning requirements may need additional dedicated ML platforms.
15. Hortonworks Data Platform
Hortonworks Data Platform (HDP) is an open-source data management and analytics platform specializing in big data management and processing. HDP is built upon Apache Hadoop, an open-source framework for distributed storage and processing of large datasets. Key features of HDP include Hadoop Distributed File System (HDFS) for scalable data storage, Apache Hive for SQL-based querying, and Apache Spark for data processing. HDP provides tools for data integration, governance, and security, supporting various data formats, including structured and unstructured data. HDP runs on commodity hardware and offers deployment flexibility, allowing on-premises or cloud deployment for data management.
Pros
Hortonworks Data Platform offers several benefits. Its open-source nature provides access to a vibrant community and a wide range of third-party integrations. HDP’s scalability enables efficient processing and storage of large datasets, making it suitable for big data analytics. The platform supports SQL-based querying with Apache Hive, reducing the learning curve for SQL-savvy users. HDP features data governance capabilities, including metadata management and access control, essential for data security and compliance. Its deployment flexibility allows organizations to choose the environment that aligns with their needs.
Cons
HDP’s open-source nature means organizations may need to invest in additional support and expertise for effective platform maintenance and management. The Hadoop ecosystem, while powerful, can be complex to configure and optimize, demanding skilled administrators and data engineers. HDP’s resource-intensive nature may necessitate significant hardware resources, potentially increasing infrastructure costs. Users accustomed to traditional relational databases may find transitioning to HDP and its non-SQL-based tools challenging. As the big data landscape evolves, some organizations may prefer more streamlined and user-friendly solutions.