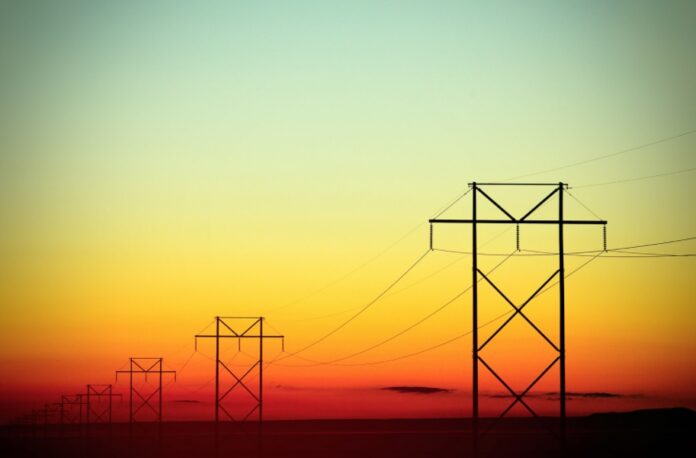
The electric utility sector has great potential to embrace artificial intelligence (AI) in the coming years. Machine learning, robotics, and automation can help electric utilities better predict supply and demand, balance the grid in real-time, reduce downtime, maximize yield, and improve the end-user experience at every step of the value chain, from power generation to end consumers.
The increasing deployment of renewable energy sources has introduced significant volatility in energy supply, with a variation of up to 60 percent. Demand fluctuates dramatically and frequently by time and region, with weather and events such as the Super Bowl creating demand peaks sometimes for less than an hour.
Following a wave of investment in the 1970s and 1980s, transmission and distribution companies have put far less money into improving their networks in the decades due to financial constraints and regulatory requirements to keep costs and rates low. As a result, today’s energy grids are ill-equipped to smooth out these spikes, and surplus power is routinely sold at a premium.
Artificial intelligence and robotics are gaining traction due to an increasingly complex network of stakeholders and assets, aging critical capital assets, highly uncertain demand and supply, non-linear power loads, cost pressures, and price deregulation.
Ultra-accurate forecasts
Demand and supply forecasting is one of the most pressing AI applications in the energy sector. Many stakeholders are affected by an inaccurate load forecast in the power grid: power generation allocates power sources for the next 24 hours based on one to two-day forecasts; transmission grids assign resources based on power transmission requirements; electricity retailers calculate energy prices on estimated demand. Electric utilities are beginning to investigate artificial intelligence technologies to produce more accurate short-term load forecasts, reduce energy consumption, and maximize the use of renewable energy despite its inconstancy.
In the future, machine and deep learning technologies could forecast demand and supply in real-time and optimize load dispatch, thereby saving energy and cost. Savings of 100 megawatts over one to four hours per day could be achieved on a network with demand ranging between 10 and 18 gigawatts. More accurate forecasts would allow utilities to postpone or avoid the construction of a fossil-fuel-powered plant. It would also provide operators with cost-effective alternatives to building new plants to accommodate seemingly impossible variability.
Yield optimization, predictive outage, and preventive maintenance
The other lever where AI and robotics could help reduce costs is operations, from power generation to transmission and distribution. Power companies could improve their generation efficiency by making real-time adjustments across assets with AI. Machine learning, for example, can help wind turbine yields be optimized based on past performance, real-time communication with other wind farms, grid status, and wind speed and direction changes.
Reduced downtime and improved preventive maintenance can also help boost power generation yield. To date, preventive maintenance efforts have had a limited impact because firms can be overwhelmed by the sheer volume of sensor data and inaccurate alerts. This is an opportunity for AI technologies, which thrive on mountains of information.
Advanced analytics already demonstrate the benefit of intelligent maintenance. Some coal power plants, for example, could predict the timing of failures with 74 percent accuracy six to nine months in advance. AI applications could increase power generation earnings before interest, taxes, depreciation, and amortization (EBITDA) by 10 to 20% by optimizing preventive maintenance, automating fault prediction, and increasing capital productivity.
Machine learning can significantly reduce energy losses in transmission and distribution. AI can make intelligent, real-time decisions to balance the grid by looking across multiple assets on a customer site, such as rescheduling the consumption of power assets and leveraging the inherent flexibility of air conditioning and refrigeration systems to maximize the benefit of solar panels and batteries installed on site.
By shaving off demand peaks during the day, utilities could postpone or even forgo the need to add generating capacity that would be required—and would generate revenue—only for short periods. Transmission and distribution firms also could shift from time-based maintenance to condition-based maintenance. Leveraging data from sensors, communications devices, and other hardware that tracks and controls objects remotely, machine learning applications could liberate grid operators from decommissioning assets before their useful lives have ended while enabling them to perform more frequent inspections and maintenance to keep assets working well.
In the future, one can imagine that operational trade-offs among several power stations or within the distribution network—such as outage planning refinement, replacement vs. run-to-failure decisions, and cutoff for spare delivery—will be automatically made by advanced analytics and machine learning algorithms. When necessary, inspections could be automatically scheduled, with machine learning algorithms making a judgment call on whether the deployment of drones and smart robots could be sufficient or whether human intervention is needed right away. For example, drone-based surveillance can replace turbines’ time-intensive and risky manual inspection. This reduces turbine downtime because inspections by drones or robots can happen while turbines remain running, helping keep costs down.
Optimization of electricity tariffs
Machine learning applications can tailor electricity prices based on the large amount of data generated by the growing number of smart meters and other devices and sensors. If regulators allow dynamic tariffs in the future, utilities may be able to use machine learning-based dynamic pricing to protect margins and reduce customer churn while maximizing assets. They could, for example, use time-of-day pricing to encourage customers to shift non-essential consumption to times when demand is lower, such as earlier in the morning or later in the evening.
Energy retailers could also use AI to create custom benefits for their most valuable, high-volume customers, such as lower rates or extra service. While price sensitivity is an important factor in attracting new customers and reducing churn, machine learning can help utilities identify the most profitable customers.
The rise of smart grids worldwide also opens the door for AI to help with energy trading, not just for utilities but also for “prosumers,” or consumers who will be able to sell excess power back to grid operators. Data and analytics are changing how markets connect sellers and buyers for various products and services, especially grid operators.
Better user experience
Energy consumers also can benefit from AI. Since the liberalization of energy retailing, new entrants have piled into the market. In Europe, customers can choose from more than 20 suppliers, competing on price alone. AI can help understand consumption patterns, tailor the value proposition and consumption to the users’ preferences, and limit the hurdles for switchers.
Machine learning can help consumers deal with the complex task of selecting their electricity supplier based on users’ preferences regarding pricing energy generation type and metering measurements. In the future, AI could automatically switch energy plans without consulting consumers or interrupting service, as the best deals become available for that specific user’s profile.
Consumers could also benefit from detailed real-time insights on their energy consumption. Machine learning applied to metering data could extract energy profiles of the home’s largest appliances and see how much each device contributes to the electricity bill. Users could also benefit from tailored consumption management. AI-enabled devices can identify heating usage patterns and learn about inhabitants’ preferences to tailor heating regulations.
Another area where AI can help improve the user experience is customer service. Virtual agents offer the opportunity to reduce customer service costs. Some retail utilities are already testing virtual agents to answer consumers’ queries and provide instant help, using natural language to understand consumers’ comments and machine learning to help customers deploy and use their power. The advancement of natural language technologies will eventually allow customer service to be fully automated.